The One Sample T Test
Compare a mean of one sampled group with some hypothesized mean or the mean of a population.
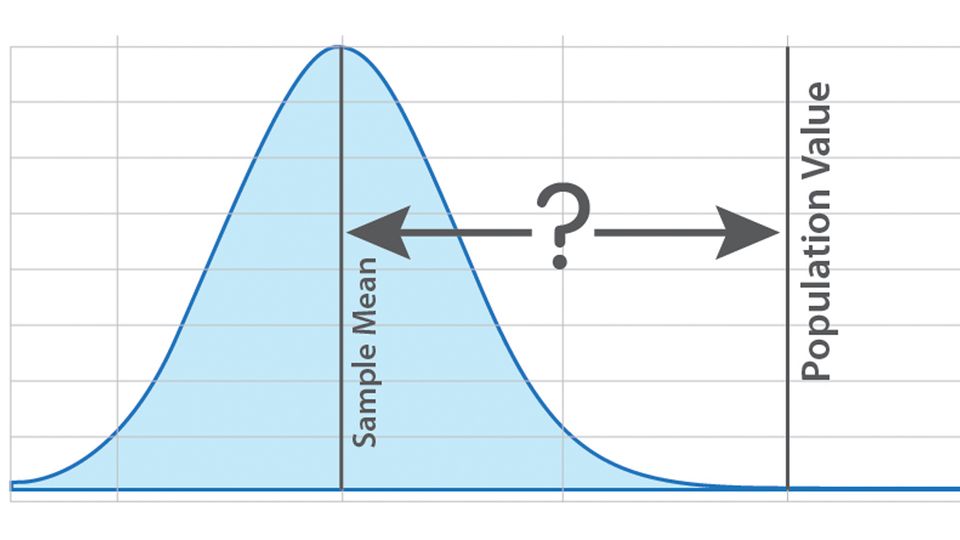
Complete the form below to unlock access to ALL audio articles.
A common objective in applying a statistical test is to compare the mean (average) of one sampled group with some hypothesized mean or the mean of a population. For example, you might want to compare the average weight of a study group with a national average or average from a wider population. The one sample t test is a good approach to answer such questions, representing a useful and flexible tool for drawing conclusions about sampled data when the variable is continuous. In this article, we will explore some of the theory behind the one sample t test, assumptions of the test, interpretation and a worked example.
What is a one sample t test?
One sample t test formula
What are the assumptions of the one sample t-test?
T test vs z test
When to use a one sample t test
One sample t test interpretation
One sample t test example
What is a one sample t test?
The t test is a commonly used hypothesis test in statistics that allows us to compare the mean value of a group of sampled data with some hypothesized value, usually a population mean value (from the same population or a different population). Since the test compares means, the variable of interest should be continuous (for example, age or weight).
There are two distinct types of t test: the one sample t test and two sample t test. The two sample t test compares two groups with respect to their mean values, whereas the one sample test compares one group with a hypothesized value. This article will focus on the one sample t test.
The test hypotheses are as follows:
- Null hypothesis (H0) is that the mean value of a group of sampled data is equal to the hypothesized value, usually a population mean value (from the same population or a different population) (µ = µ0)
- Alternative hypothesis (H1) the mean value of a group of sampled data is not equal to the hypothesized value, usually a population mean value (from the same population or a different population) (µ ≠ µ0)
One sample t test formula
The formula to calculate the test statistic t, or t-value, for the one sample t test is as follows:

Where x̄ is the mean value in the sample, μ is the population or hypothesized mean and sx̄ is the sample's standard error of the mean. The standard error sx̄ can be found using the following formula:

Where s is the sample's standard deviation and n is the number of observations in the sample.
What are the assumptions of the one sample t-test?
Assumptions for the one sample t test are as follows:
- The data in the variables of interest should be continuous. This means they take any numeric value, such as age in years, weight in kilograms or exam performance (0–100).
- The data should be drawn from a random sample of the population we are trying to make an inference about. This is to ensure the data are representative of this population and that the inference made from the test is valid.
- The data should be independently sampled, meaning that observations are not related to each other.
- The data in the variable of interest should be approximately normally distributed. It should be noted that the one sample t test is relatively flexible to departures from this assumption, so that if it deviates from a Normal distribution partially, then the results may still be valid.
T test vs z test
The t test and the z test share a key similarity in that they are both used to compare means between one or two groups to make inferences about how these values differ. As with the t test, there is both the one sample and two sample z test available depending on the hypotheses being investigated.
It is important to note that the z test is not often used in practice because it requires the population standard deviation to be known and this is rarely the case. The standard deviation is a statistical measure that quantifies the spread (or variability) of the data around the mean value. By contrast, the t test uses a sample estimate of the standard deviation. The z test is usually used to demonstrate statistical theory for hypothesis testing. Another key difference is that the z test uses the Normal distribution to derive the test’s critical value whereas the t test uses the t-distribution. The t-distribution is another common probability distribution in inferential statistics, a key characteristic of which is that it changes as sample size changes.
When to use a one sample t test
The one sample t test should be chosen when one sample is available and an investigator would like to make a comparison between the mean value of a continuous variable in that sample with a population mean value (either real or hypothesized). If the hypothesis of interest is whether there is a difference between the means of two sampled groups, then the two sample t test should be used instead. Moreover, if the samples are paired, then the paired samples t test should be used.
If the sample size is small (usually less than 15 is used as a rule of thumb), it can no longer be assumed that the data take a Normal distribution and so a non-parametric test such as the one-sample Wilcoxon signed rank test should be used instead. To test differences between means in more than two samples, an analysis of variance (ANOVA) should be used.
One sample t test interpretation
Interpreting the one sample t test will allow us to make an inference about whether a sample differs from a population or hypothesized mean value. There are two distinct interpretations depending on which p-value is derived, whether it is a one- or two-tailed test. The two-sided p-value (from the two-tailed test) is usually of more interest as it gives both directions of the effect. In other words, it would interpret as to whether there is a difference (in any direction) between the sample mean and hypothesized mean. The one-tailed test would interpret as to whether the sample mean is larger (or smaller) than the hypothesized mean.
One sample t test example
Suppose a clinical audit of 20 hospitals was conducted, a care quality score (0–100) was calculated for each and researchers were interested in investigating whether these hospitals differ from a national average quality score (Figure 1). The researchers opt to compare the mean quality score with a national average using the one sample t test. The mean value for the 20 hospitals audited is x̄ = 84.5, the standard deviation is s = 5.2 and the national average is μ = 90.3. In practice, statistical software can easily calculate the one sample t test to compare these means, but below are the steps to conducting the test by hand.
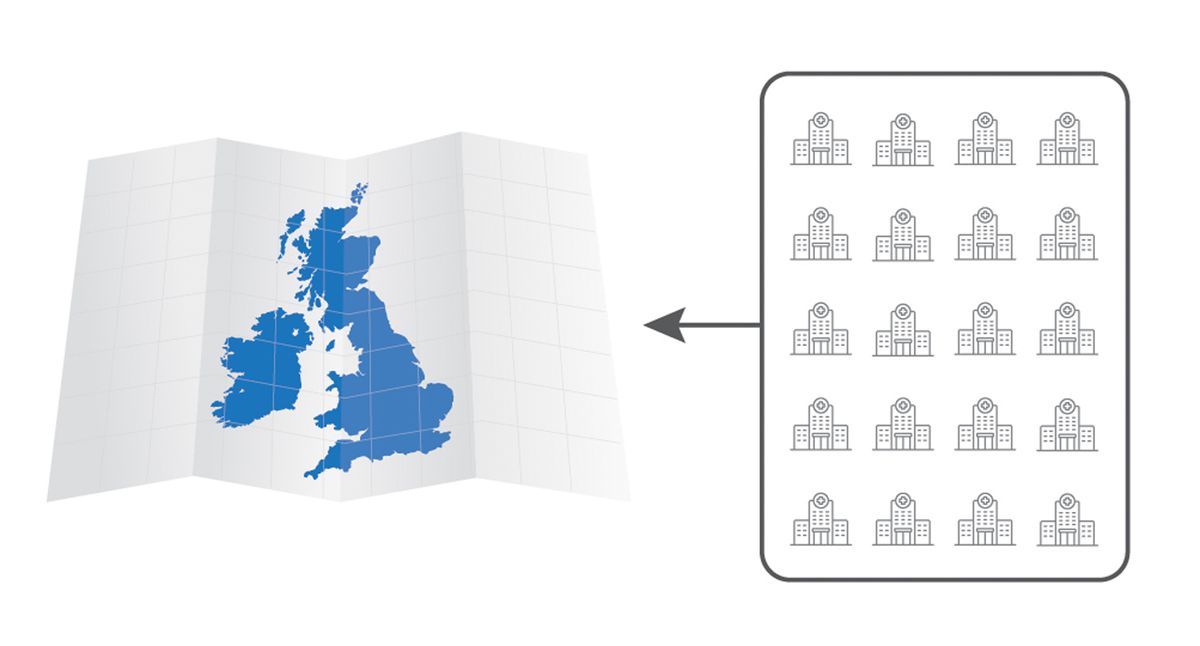
Figure 1: A sample of 20 hospitals are compared to a national average for their care quality score.
- The null hypothesis is that the audited hospital quality scores are the same as the national average score.
- The alternative hypothesis is that the audited hospital quality scores differ from the national average score.
First, the standard error of the mean can be calculated:
Next, the one sample t test statistic can be calculated:
Given our test statistic (t = 6.66), we can now use a t table to find the critical value at the relevant t value and degrees of freedom (df). Df is the number of independent bits of information in a statistical model or test, which informs the critical value and p-value. In this case, our df value can be calculated as df = n – 1 = 20 – 1 = 19. By reading the df = 19 row in the t table, we can see that our test statistic value is larger than 3.883 (the last column). We then read off the column value and conclude that the p-value (1 – 0.999) is p < 0.001 under a two-tailed test. Based on this small p-value, we can reject the null hypothesis and conclude that there is a difference in quality scores between the hospitals audited and the national average and that there is strong evidence the difference is unlikely to be due to chance.
Further reading:
- Bland M. An Introduction to Medical Statistics (4th ed.). Oxford. Oxford University Press; 2015. ISBN:9780199589920
- DATAtab. One sample t-test. https://datatab.net/tutorial/one-sample-t-test, DATAtab, Accessed 16 May 2024.
- Laerd Statistics. One-sample t-test using SPSS statistics. Laerd Statistics, https://statistics.laerd.com/spss-tutorials/one-sample-t-test-using-spss-statistics.php, Accessed 20 May 2024.