Implementing AI in Drug Discovery
Explore the practical considerations of enhancing your drug discovery workflows with artificial intelligence methods.
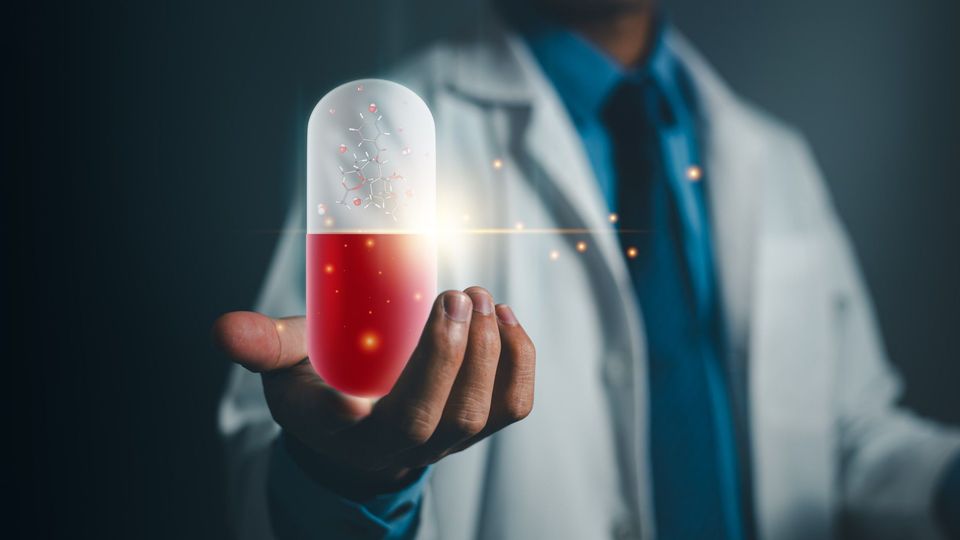
Complete the form below to unlock access to ALL audio articles.
Over the past few years, the industry has been abuzz with sensationalist headlines about how drug discovery will be revolutionized by artificial intelligence (AI), allowing the perfect drug candidates to be created in mere months. The opposite is also true, with headlines citing risks from unsubstantiated methods and false AI claims. Is AI all just hype without any real impact? Or perhaps AI is too powerful, with the potential to replace chemists?
The truth is that while research in this booming field has resulted in exciting new algorithms, in practice, there’s a lot that needs to be done to harness AI to its fullest potential in drug discovery. The four main considerations that any leader should take into account to successfully implement AI in their organization are explored here.
Purpose and power: the AI algorithms
The starting point is an AI algorithm, which must do something useful. What do you want to achieve with AI? Alongside more general applications like literature collation or writing support and biology-focused applications around data analysis, there are three main areas in which AI can provide a substantial impact on drug discovery chemistry. These are generative chemistry, predictive modeling and retrosynthetic analysis. So, what must AI achieve in these fields to actually be useful?
It’s easy to generate arbitrary new chemical structures by applying AI in generative chemistry. However, to be useful, AI-derived compounds must also be chemically meaningful, stable and synthesizable. On top of this, the suggestions must be relevant to the project objectives, including activity against specific targets and appropriate physicochemical and ADMET properties.
Synthetic feasibility carries over into retrosynthetic analysis. Does the AI provide realistic suggestions for synthesizing complex molecules? Can an AI generalize beyond the reactions with which it has been trained to suggest novel routes? And, of the routes suggested, are any of these useful for industrial scale-up and synthesis in large quantities?
For predictive modeling, the AI must recognize when it can make accurate predictions, or, conversely, where it has greater uncertainty and more experimental data are needed. Only with this information can decision makers rely on predictive models to confidently guide compound or experiment prioritization.
Providing proof points
While it may be relatively easy to achieve positive results against artificial metrics, it is much more difficult to make a substantial difference to drug discovery projects on a reproducible basis. To establish the benefits, the algorithm must be applied to a diverse range of projects and different data sets. Ideally, one would run multiple iterations of experiments, both harnessing the AI and without it, to achieve statistical significance; however, time and resource limitations mean the initial evidence of success may need to be on a smaller scale.
Compelling case studies and examples can act as evidence, but these should be carefully considered. Some of the poor reputation of AI comes down to examples of publicized case studies claiming to have progressed from hit-to-candidate in months.
However, when examined, we can see that the drug targets involved are well-characterized with lots of related data, presenting an ideal scenario for any AI algorithm. This scenario is completely unrealistic when compared to most real drug discovery programs, where initial data sets are sparse and contain high levels of uncertainty and inaccuracy. Therefore, these idealized case studies provide limited proof of success.
Only strong evidence will overcome the widespread skepticism about AI and lead to routine adoption of methods that can make a valuable contribution to drug discovery.
Easy access for widespread adoption
In addition to proven effectiveness, algorithms must also be easily applied. It’s all very well for an AI method to work when carefully nurtured by an AI expert, but this will create a bottleneck and limit its impact, unless it can be applied directly by scientists with different expertise across drug discovery project teams. However, successful deployment can often take more work than developing the initial algorithm.
The challenges to this are threefold: robustness, scalability and accessibility. The implementation must work regardless of what a user throws at it; it can’t rely on code being tweaked to deal with every special case. The platform must be able to handle realistic data sets and routine use simultaneously by many users, and the results that come back must be easily interpretable and the implications for decisions clear.
Traditionally, AI has sat with small groups of computational chemists and data scientists within drug discovery institutes. However, there aren’t enough of these experts to support even a fraction of ongoing drug discovery projects. Accessible, intuitive access through user-friendly interfaces is essential to broaden AI’s user base. An “augmented intelligence” approach to drug discovery chemistry enables scientists to enhance their decision-making by complementing their expertise with powerful AI methods to achieve the maximum impact.
An open mind at the organizational level
The final piece to the puzzle to gain the greatest benefit from AI is around organizational change. This doesn’t mean replacing jobs with AI or hiring an army of computational experts to support implementation, this is about embracing different tasks and workflows to maximize the value of the AI you are using.
AI requires relevant data to recognize patterns and make predictions and suggestions. This may mean synthesizing compounds you wouldn’t have considered based on medicinal chemistry training or conducting experiments which, on the surface, may not provide immediate obvious value. It’s about feeding the AI with the information it needs so that, in future, the algorithm will be able to guide compound, assay or experiment prioritization more effectively.
By adapting our way of working to implement AI, chemists will be able to cut through the hype and gain enormous value from these new technologies.
Reaping the benefits
Purpose, proof, deployment and adaptation. With these four considerations, the value we can extract from AI methods will significantly increase. Some may consider this prospect with trepidation, but it’s something to be embraced, not feared. After all, AI will not replace skilled chemists. However, chemists who embrace AI may replace those who do not.
About the author:
Dr. Matthew Segall has a Master of Science in computation from the University of Oxford and a PhD in theoretical physics from the University of Cambridge. He has led teams developing predictive models and intuitive decision-support and visualization tools for drug discovery. In 2009 he led a management buyout of the StarDrop business to found Optibrium. Optibrium develops innovative software and AI solutions to improve the efficiency and productivity of drug discovery, such as Cerella™, a deployable AI platform that enables research teams to go from drug concept to candidate faster, pinpointing the best compounds for synthesis and guiding decision-making by revealing transformative insights from sparse, complex discovery data.